Data in Motion: Understanding Data Aggregation and Anonymisation in the Context of Transportation
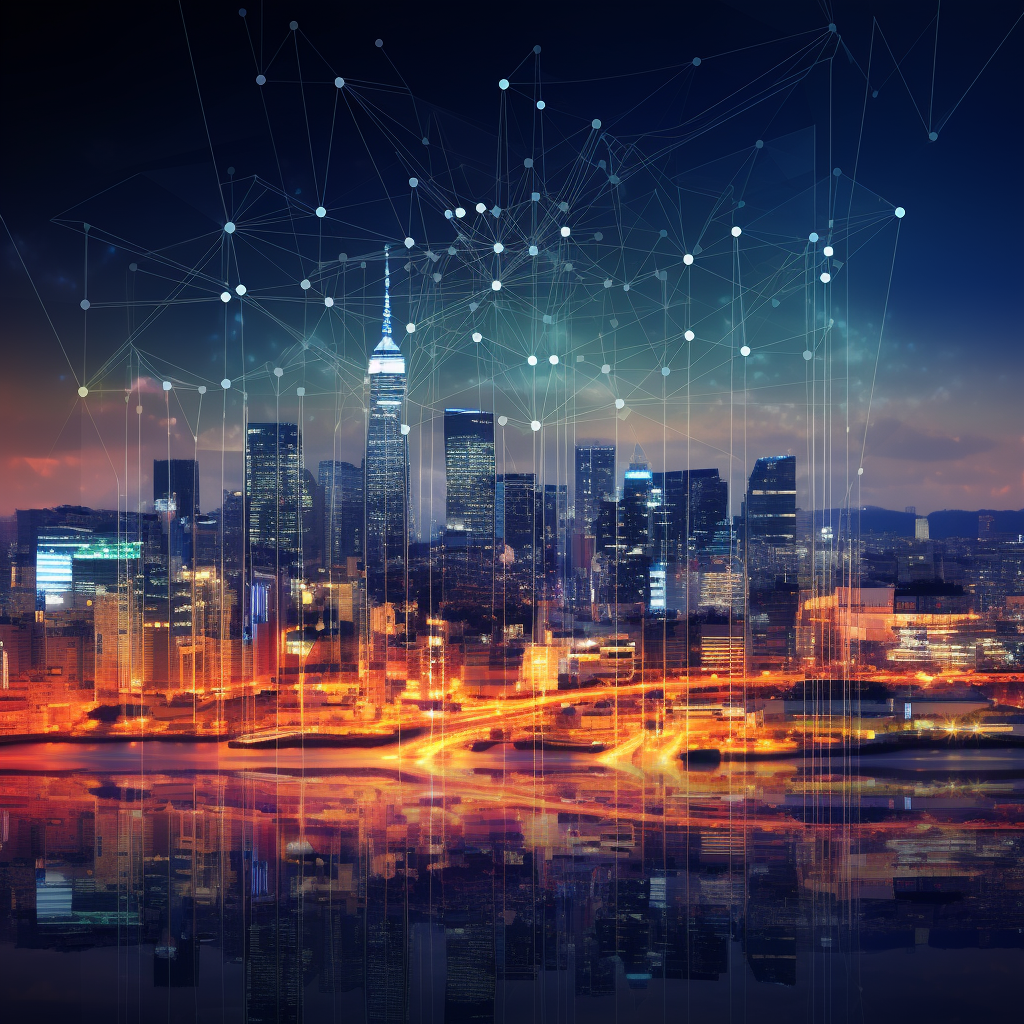
Data Aggregation: From Individual Trips to Global Patterns
Data aggregation is the process of combining individual data points to form a comprehensive, summarised view. This could involve gathering data from millions of individual vehicle movements to understand larger traffic trends and patterns.
For example, a telematics company might collect data on vehicle speeds, routes, and times for thousands of cars. While the specific data for each car might be interesting, the real value comes from aggregating this data to observe broader patterns. This could reveal rush hour peaks, frequent traffic bottlenecks, popular commuting routes, or areas lacking connectivity, which is crucial information for city planners and transport policymakers.
However, this wealth of information comes with a catch: It's only possible to aggregate this data by collecting detailed, individual-level information, raising privacy concerns.
Anonymisation: Securing Privacy
To address these privacy issues, transportation researchers and companies employ anonymisation, removing or obscuring personal identifiers from data sets to protect individual privacy. This means removing details that could identify an individual's travel patterns for transportation data.
For instance, consider a city's public bike-sharing program. Users unlock bikes using an app, which records data such as where each journey starts and ends, the duration of the trip, and the route taken. However, knowing someone's daily routes could reveal sensitive information, like their home and work locations or their children's school.
To avoid this, the program's operator can anonymise the data by removing or changing personally identifiable information (PII). They could use wider geographic zones instead of precise start and end locations. Exact trip times could be replaced with time bands (e.g., morning, afternoon, evening). These steps ensure the data can provide useful insights about usage patterns without risking user privacy.
Striking the Right Balance: The Challenges of Aggregation and Anonymisation
The delicate balance between data utility and privacy forms a central challenge in dealing with transportation data. Aggregated, anonymised data should be insightful and non-invasive, but getting this balance right is often difficult.
A notable case is New York City's Taxi and Limousine Commission, which 2014 released an anonymised dataset detailing over 173 million individual taxi trips. Although the data was anonymised, researchers found it possible to re-identify drivers and passengers by combining this dataset with auxiliary information, such as photos of taxi medallion numbers around the city.
This case underscores a critical concern with anonymisation: It can sometimes be undone if not done meticulously. A robust anonymisation process must consider potential 'back doors' and ensure the data cannot be de-anonymised, even when combined with other data sets.
Navigating the Future of Transportation Data
Telematics and other data-driven technologies will be increasingly integral as the transportation sector evolves. Data aggregation and anonymisation provide powerful tools for deriving valuable insights while safeguarding individual privacy. However, as we've seen, these processes come with significant challenges that necessitate rigorous methodologies and thoughtful regulations.
Understanding these processes is vital not just for researchers, transportation officials, and privacy advocates but also for everyday citizens. After all, it is our trips that populate these datasets and our cities that are shaped by these analyses. The balance between utility and privacy in transportation data is not just a technical issue; it's a social contract for the digital age that requires ongoing engagement and understanding.